粒子滤波(Particle Filter)是一种基于蒙特卡罗方法的滤波技术,用于非线性、非高斯系统的状态估计。上期叙述了粒子滤波的理论与公式。本文将分析粒子滤波的实现过程,并通过一个简单的一维非线性系统的例子来展示其应用。
首先,我们定义了一个简单的非线性系统模型,具体形式为:
x
k
=
0.5
?
x
k
?
1
+
25
?
x
k
?
1
1
+
x
k
?
1
2
+
8
?
cos
?
(
1.2
?
u
k
)
+
?
k
\begin{equation} x_k = 0.5 \cdot x_{k-1} + \frac{25 \cdot x_{k-1}}{1 + x_{k-1}^2} + 8 \cdot \cos(1.2 \cdot u_k) + \epsilon_k \end{equation}
xk?=0.5?xk?1?+1+xk?12?25?xk?1??+8?cos(1.2?uk?)+?k???
其中,
x
k
x_k
xk?) 是系统在时刻
k
k
k的状态,
u
k
u_k
uk?是输入,
?
k
\epsilon_k
?k? 是系统噪声。
粒子滤波通过一组粒子来近似系统状态的概率分布。在我们的例子中,粒子滤波函数的主要步骤包括:
我们将粒子滤波应用于一个一维非线性系统,通过生成真实状态和带有噪声的观测数据,然后利用粒子滤波来估计系统的状态。通过调整粒子数量和初始状态猜测,我们可以观察粒子滤波在估计过程中的表现。
下面是通过 Python 实现的粒子滤波代码:
# @copyright all reseved
# @author: Persist_Zhang
import numpy as np
import matplotlib.pyplot as plt
# 定义非线性系统模型
def nonlinear_system_model(x, u):
# 这里以一个简单的非线性系统为例,可以根据实际情况修改
return 0.5 * x + 25 * x / (1 + x**2) + 8 * np.cos(1.2 * u)
# 粒子滤波函数
def particle_filter(num_particles, measurements, initial_state):
particles = np.random.randn(num_particles) + initial_state
weights = np.ones(num_particles) / num_particles
estimated_states = []
for measurement in measurements:
# 递推状态预测
particles = nonlinear_system_model(particles, 1) + 0.1 * np.random.randn(num_particles)
# 权重更新
likelihood = np.exp(-0.5 * (particles - measurement)**2)
weights *= likelihood / np.sum(likelihood)
# 归一化权重
weights /= np.sum(weights)
# 重采样
indices = np.random.choice(np.arange(num_particles), size=num_particles, replace=True, p=weights)
particles = particles[indices]
# 估计结果
estimated_state = np.sum(weights * particles)
estimated_states.append(estimated_state)
return estimated_states
# 生成真实状态数据
np.random.seed(42)
true_states = []
for _ in range(100):
true_states.append(nonlinear_system_model(true_states[-1] if true_states else 0, 1) + 0.1 * np.random.randn())
# 生成观测数据
measurements = true_states + 0.5 * np.random.randn(100)
# 初始化粒子滤波
num_particles = 1000
initial_state_guess = 0
estimated_states = particle_filter(num_particles, measurements, initial_state_guess)
# 绘制结果
plt.figure(figsize=(10, 6))
plt.plot(true_states, label='True States', color='blue')
plt.scatter(range(len(measurements)), measurements, label='Measurements', color='red', marker='x')
plt.plot(estimated_states, label='Particle Filter Estimate', color='green')
plt.legend()
plt.xlabel('Time')
plt.ylabel('State')
plt.title('Particle Filter for Nonlinear System')
plt.savefig('./ParticleFilterng')
plt.show()
```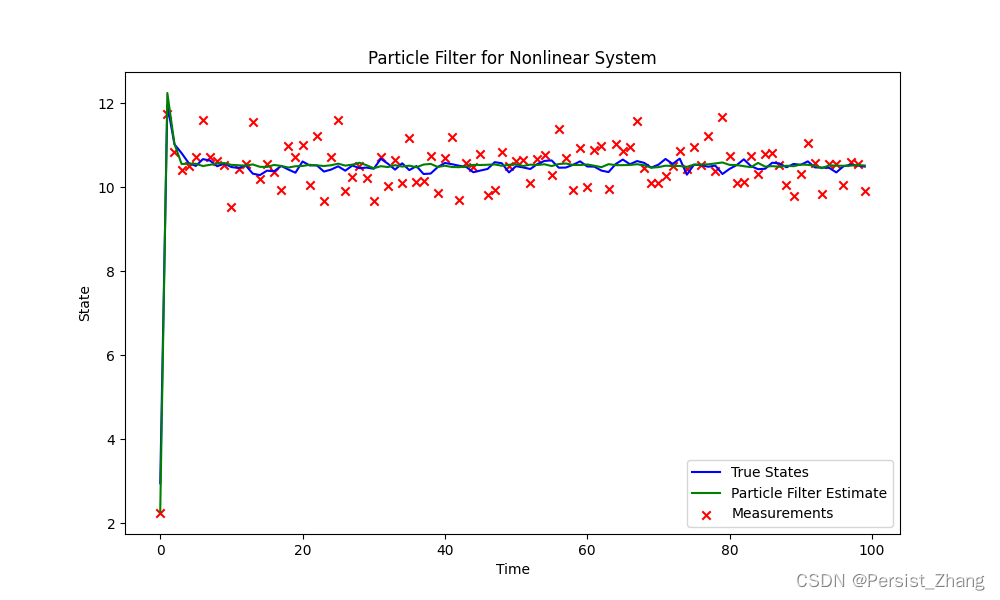
通过运行上述代码,我们可以观察到真实状态、观测数据以及粒子滤波的估计结果。这张图展示了粒子滤波在非线性系统中的应用,它通过考虑不确定性和噪声,对系统状态进行了有效的估计。
## 结论
粒子滤波作为一种非参数化、适用于非线性系统的滤波方法,为处理实际应用中的复杂问题提供了有力的工具。通过合理设置粒子数量和初始条件,粒子滤波可以在非线性系统中提供较为准确的状态估计。然而,也需要注意到其计算复杂度随粒子数量增加而增加的特点,因此在实际应用中需要权衡计算资源和精度。