前言
本文接上一篇文章《斯坦福机器人Mobile ALOHA的关键技术:动作分块ACT的算法原理与代码剖析》而来,当然最开始本文是作为上一篇文章的第二、第三部分的
但因为ACT太过关键,除了在上一篇文章中写清楚其算法原理之外,还得再剖析其代码实现,故为避免上一篇文章太过长,也为更清楚的阐述Diffusion Policy、VINN,故便有了本文
第一部分?Diffusion Policy
如我组建的复现团队里的邓老师所说,斯坦福mobile aloha团队也用了 diffusion,不过是作为对比实验的打击对象来用的
下面,我们便根据Columbia University、Toyota Research Institute、MIT的研究者联合发布的《Diffusion Policy:Visuomotor Policy Learning via Action Diffusion》这篇论文详细解读下Diffusion Policy
1.1 什么是Diffusion Policy
如下图所示
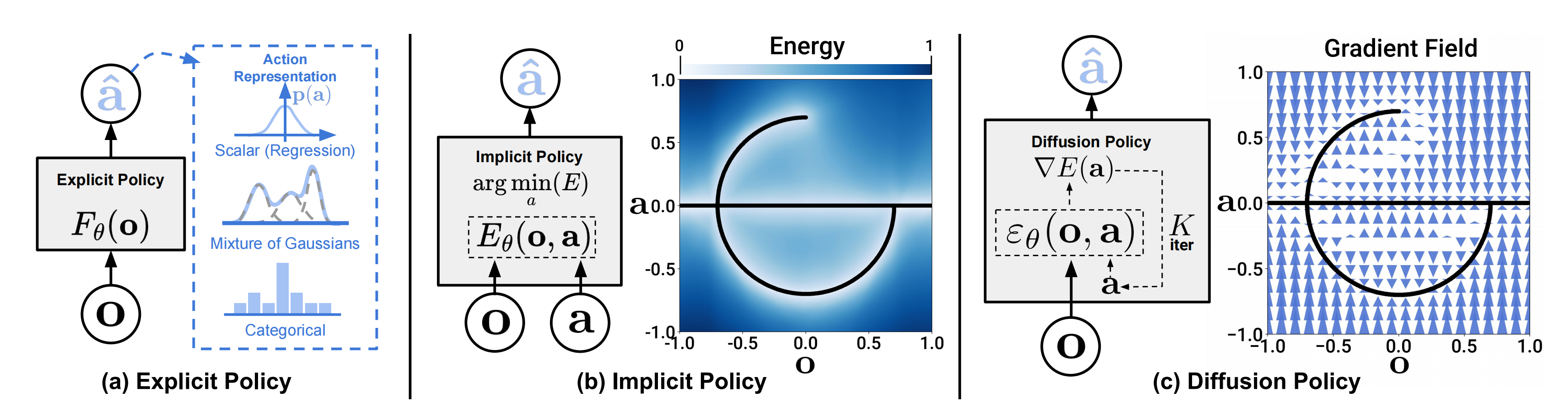
- a)具有不同类型动作表示的显式策略(Explicit policy with different types of action representations)
- b)隐式策略学习以动作和观察为条件的能量函数,并对最小化能量景观的动作进行优化(Implicit policy learns an energy functionconditioned on both action and observation and optimizes for actions that minimize the energy landscape)
- c)扩散策略通过学习的梯度场将噪声细化为动作。这种表述提供了稳定的训练,允许学习到的策略准确地建模为多模态动作分布,并容纳高维动作序列
Diffusion policy refines noise into actions via a learned gradient field. This formulation provides stable training, allows the learned policy to accurately model multimodalaction distributions, and accommodates high-dimensional action sequences
进一步,所谓扩散策略,是指将机器人的视觉运动策略表示为条件去噪扩散过程来生成机器人行为的新方法
- 扩散策略学习动作-分布评分函数的梯度
即该策略不是直接输出一个动作,而是以视觉观察为条件,对K次去噪迭代推断“动作-得分梯度”(instead of directly outputting an action, the policy infers the action-score gradient, conditioned on visual observations, for K?denoising iterations) - 并在推理过程中通过一系列随机朗之万动力学步骤对该梯度场进行迭代优化。扩散公式在用于机器人策略时产生了强大的优势,包括优雅地处理多模态动作分布,适合高维动作空间,并表现出令人印象深刻的训练稳定性
- 为了充分释放扩散模型在物理机器人上进行视觉运动策略学习的潜力,作者团队提出了一套关键的技术贡献,包括将后退视界控制、视觉调节和时间序列扩散transformer结合起来
1.2?Diffusion for Visuomotor Policy Learning
如下图所示
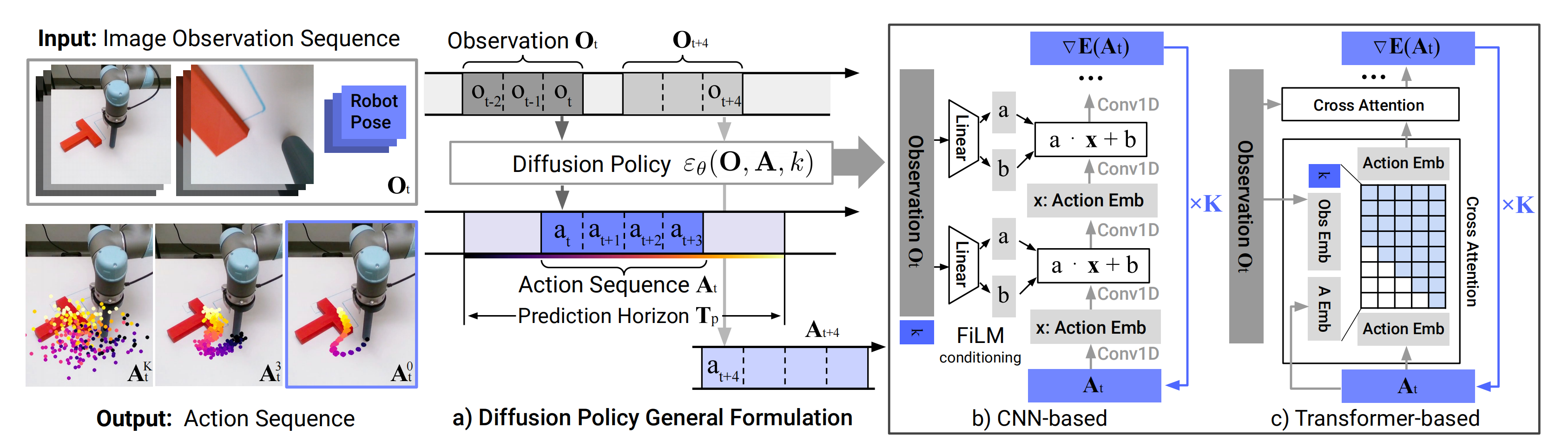
- a)一般情况下,该策略在时间步长
时将最新的
步观测数据
作为输入,并输出
步动作
General formulation.?At time step?t, the policy takes the latest?To?steps of observation data?Ot?as input and outputs?Ta?steps of actions?At - b)在基于CNN的扩散策略中,对观测特征
应用FiLM(Feature-wise Linear Modulation)[35]来调节每个卷积层通道。从高斯噪声中提取的
减去噪声估计网络
的输出,并重复
次,得到去噪动作序列
?「(这个过程是扩散模型去噪的本质,如不了解DDPM,请详看此文:《AI绘画能力的起源:从VAE、扩散模型DDPM、DETR到ViT/Swin transformer》」
In the CNN-based Diffusion Policy, FiLM (Feature-wise Linear Modulation) [35] conditioning of the observation feature?Ot?is applied to every convolution layer, channel-wise.?Starting from?AtK?drawn from Gaussian noise, the outputof noise-prediction network?εθ is subtracted, repeating?K?times to get?At0, the denoised action sequence.? - c)在基于Transformer的[52]扩散策略中,观测
的嵌入被传递到每个Transformer解码器块的多头交叉注意力层。每个动作嵌入使用所示注意力掩码进行约束,仅关注自身和之前的动作嵌入(因果注意)
In the Transformer-based [52]Diffusion Policy, the embedding of observation?Ot?is passed into a multi-head cross-attention layer of each transformer decoder block.?Eachaction embedding is constrained to only attend to itself and previous action embeddings (causal attention) using the attention mask illustrated.
虽然DDPM通常用于图像生成,但该团队使用DDPM来学习机器人的视觉运动策略。这需要针对DPPM的公式进行两大修改
- 之前输出的是图像,现在需要输出:为机器人的动作(changing the output x to represent robot actions)
- 去噪时所依据的去噪条件为观测Ot?(making the denoising processes conditioned on input observation Ot)
具体来说,在时间步
,该策略以最新
步的观测数据Ot作为输入,预测
步的动作。其中,机器人执行
步的动作时无需重新规划。在此定义中,
表示观测视界,
表示动作预测视界,而
则代表了动作执行视界。这样做既促进了时间动作的一致性,又保持了响应速度
我们使用DDPM来近似条件分布p(At|Ot),而不是Janner等人[20]用于规划的联合分布p(At,Ot)。这种表述方式允许模型以观察为条件来预测动作,而无需以推断未来状态的成本(This formulation allows the model to predict actionsconditioned on observations without the cost of inferringfuture states),加快扩散过程并提高生成动作的准确性
- 众所周知,从从高斯噪声中采样的
开始,DDPM执行
次去噪迭代,以产生一系列降低噪声水平的中间动作,
,直到形成所需的无噪声输出
?(说白了,就是去噪)
该过程遵循下述所示的公式1 
其中
为通过学习优化参数的噪声估计网络,
为每次迭代时加入的高斯噪声
且上面的公式1也可以理解为一个单一的噪声梯度下降步长,定义为如下公式2
????????????????????????????????????????????????
其中噪声估计网络
有效地预测了梯度场
,
为学习速率
此外,公式1中的
、
和
作为与迭代步长
相关的函数选择被称为噪声调度,可以理解为梯度下降过程中学习速率的调整策略。经证明,将
设定略小于1能够改善稳定性
再之后,训练过程首先从数据集中随机抽取未修改的样本
。对于每个样本,我们随机选择一个去噪迭代
,然后为迭代
采样一个具有适当方差的随机噪声
然后要求噪声估计网络从添加噪声的数据样本中预测噪声,如下公式3 
最小化公式3所示的损失函数也同时最小化了数据分布p(x0)和从DDPM q(x0)中提取的样本分布之间KL-散度的变分下界 - 为了获取条件分布
,将公式1修改为如下公式4 
将训练损失由公式3修改为如下的公式5 
1.2.1 视觉编码器的选型:CNN PK transformer
基于CNN的扩散策略中,采用Janner等人[21]的一维时态CNN,并做了一些修改,如下图所示
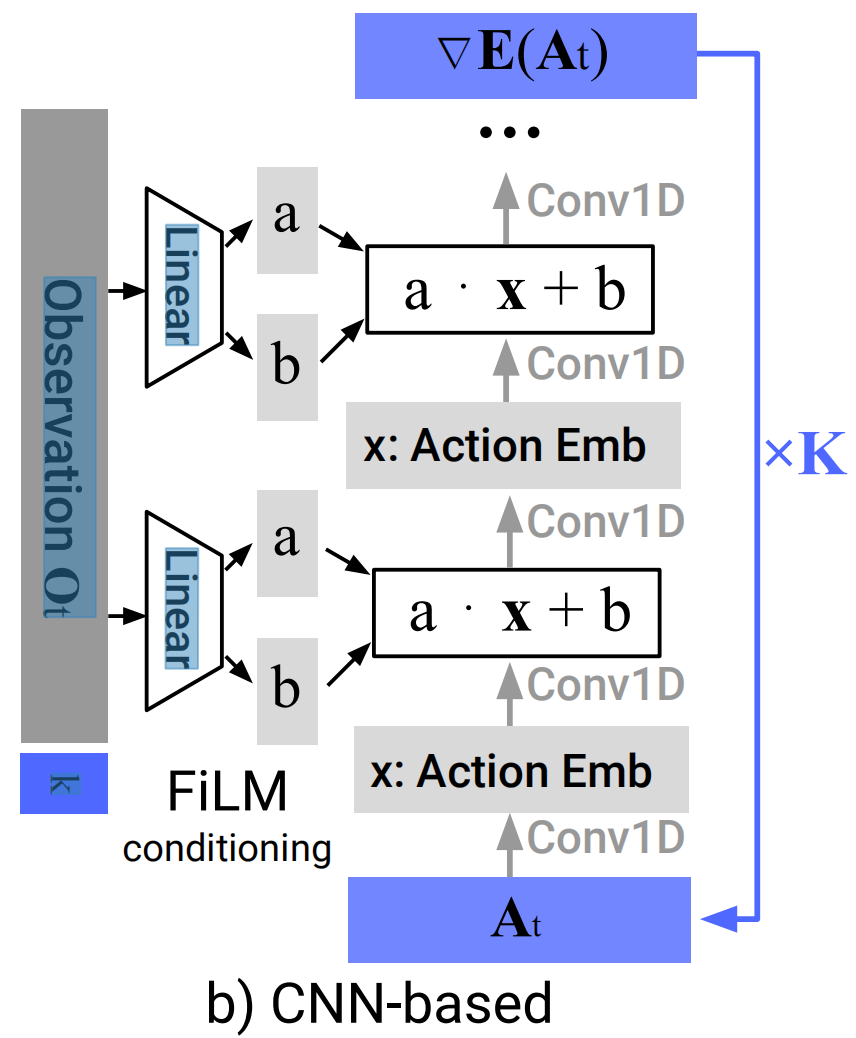
- 首先,我们仅通过特征线性调制(FiLM),和对观测特征
的动作生成过程进行调节,并进行去噪迭代
,以建模条件分布
- 其次,我们仅预测动作轨迹,而非连接观测动作轨迹(we only predict the action trajectory instead of the concatenated observation action trajectory)
- 第三,利用receding prediction horizon,删除了基于修复的目标状态条件反射。然而,目标条件反射仍然是可能的,与观测所用的FiLM条件反射方法相同
we removed inpainting-based goal state conditioning due to incompatibility with our framework utilizing a receding prediction horizon. However, goal conditioning is still possible with the same FiLM conditioning method used for observations
在实践中发现,基于CNN的骨干网络在大多数任务上表现良好且无需过多超参数调优。然而,当期望的动作序列随着时间快速而急剧变化时(如velocity命令动作空间),它的表现很差,可能是由于时间卷积的归纳偏差[temporal convolutions to prefer lowfrequency signals],以偏好低频信号所致。为减少CNN模型中过度平滑效应[49],我们提出了一种基于Transformer架构、借鉴minGPT[42]思想的DDPM来进行动作预测
如下图所示
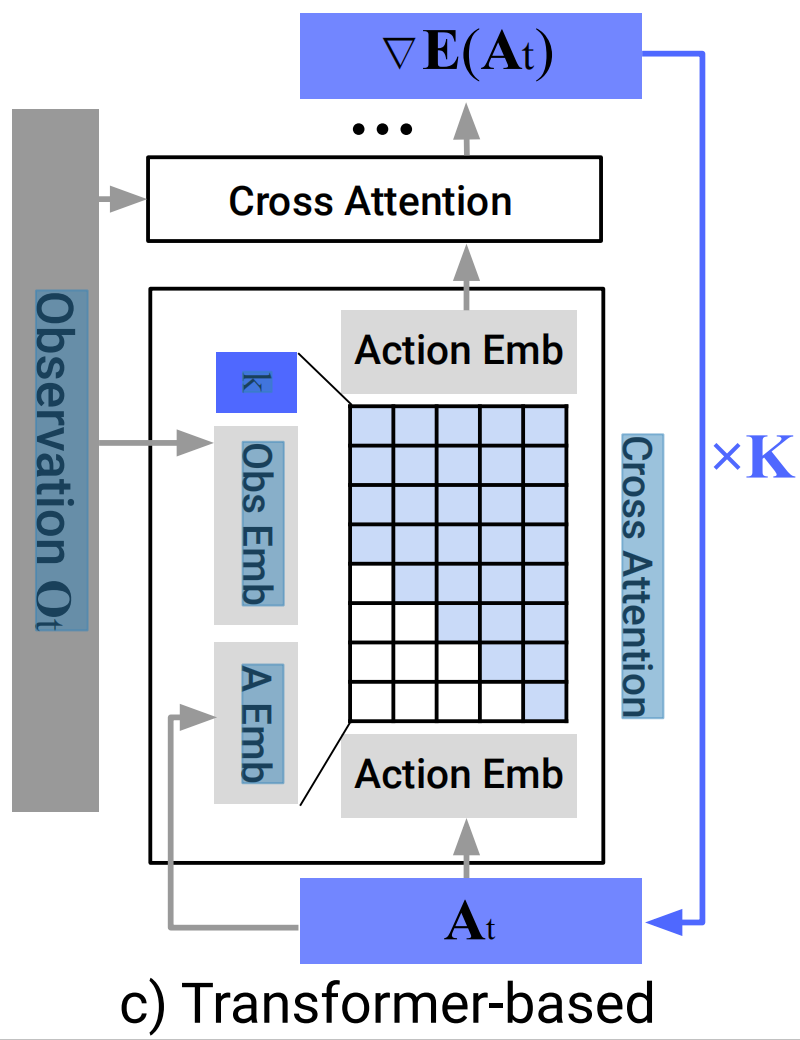
- 行动和噪声
作为transformer解码器块的输入tokens传入,扩散迭代
的正弦嵌入作为第一个token(Actions with noise At k are passed in as input tokens for the transformer decoder blocks, with the sinusoidal embedding for diffusion iteration k prepended as the first token)
观测
通过共享的MLP转换为观测嵌入序列,然后作为输入特征传递到transformer解码器堆栈中(The observation Ot is transformed into observation embedding sequence by a shared MLP, which is then passed into the transformer decoder stack as input features)
“梯度”
由解码器堆栈的每个对应输出token进行预测(The "gradient" εθ (Ot ,At k , k) is predicted by each corresponding output token of the decoder stack) - 在我们的基于状态的实验中,大多数性能最佳的策略都是通过Transformer骨干实现的,特别是当任务复杂度和动作变化率较高时。然而,我们发现Transformer对超参数更敏感
Transformer训练的困难[25]并不是Diffusion Policy所独有的,未来可以通过改进Transformer训练技术或增加数据规模来解决(However, we found the transformer to be more sensitive to hyperparameters. The difficulty of transformer training [25] is not unique to Diffusion Policy and could potentially be resolved in the future with improved transformer training techniques or increased data scale)
故,一般来说,我们建议从基于CNN的扩散策略实施开始,作为新任务的第一次尝试。如果由于任务复杂性或高速率动作变化导致性能低下,那么可以使用时间序列扩散Transformer来潜在地提高性能,但代价是额外的调优(In general, we recommend starting with the CNN-based diffusion policy implementation as the first attempt at a new task. If performance is low due to task complexity or high-rate action changes, then the Time-series Diffusion Transformer formulation can be used to potentially improve performance at the cost of additional tuning)
看到上面这里后,我第一反应是想到了此文《视频生成的原理解析:从Gen2、Emu Video到PixelDance、SVD、Pika 1.0、W.A.L.T》第六部分中提到的W.A.L.T:将Transformer用于扩散模型
“23年12月中旬,来自斯坦福大学、谷歌、佐治亚理工学院的研究者提出了 Window Attention Latent Transformer,即窗口注意力隐 Transformer,简称 W.A.L.T
该方法成功地将 Transformer 架构整合到了隐视频扩散模型中,斯坦福大学的李飞飞教授也是该论文的作者之一”
当然,既然提到了视频生成,也顺便说一嘴,如我组建的mobile aloha复现小组里邓老师所说的:“机器人比生成视频,简单太多了,例如视频由一连串图像帧构成,每一帧图像,经常是 256 * 256 * 3 个数值,而机器人只有 14 个数值”
1.2.2 视觉编码器:把图像潜在嵌入化并通过扩散策略做端到端的训练
视觉编码器将原始图像序列映射为潜在嵌入
,并使用扩散策略进行端到端的训练(The visual encoder maps the raw image sequence intoa latent embedding?Ot?and is trained end-to-end with thediffusion policy)
不同的相机视图使用不同的编码器,以对每个时间步内的图像独立编码,然后连接形成
,且使用标准的ResNet-18(未进行预训练)作为编码器,并进行以下修改:
- 使用空间softmax池化替代掉全局平均池化,以维护空间信息
1) Replace the global average pooling with a spatial softmax pooling to maintain spatial information[29] - 采用GroupNorm代替BatchNorm来实现稳定训练,在归一化层与指数移动平均(通常应用于DDPMs)结合时尤其重要
2) Replace BatchNorm with GroupNorm [57] for stabletraining.?This is important when the normalization layer isused in conjunction with Exponential Moving Average [17](commonly used in DDPMs)
1.3 扩散策略的稳定性与好处
1.3.1 动作序列预测的好处
由于高维输出空间采样困难,在大多数策略学习方法中一般不做序列预测。例如,IBC将难以有效地采样具有非光滑能量景观的高维动作空间。类似地,BC-RNN和BET难以确定动作分布中存在的模式数量(需要GMM或k-means步骤)
相比之下,DDPM在不降低模型表现力的前提下,在输出维度增加时仍然保持良好扩展性,在许多图像生成应用中已得到证明。利用这种能力,扩散策略以高维动作序列的形式表示动作,它自然地解决了以下问题:
- 时间动作一致性,如下图所示,为了将T块从底部推入目标,策略可以从左或右绕T块走
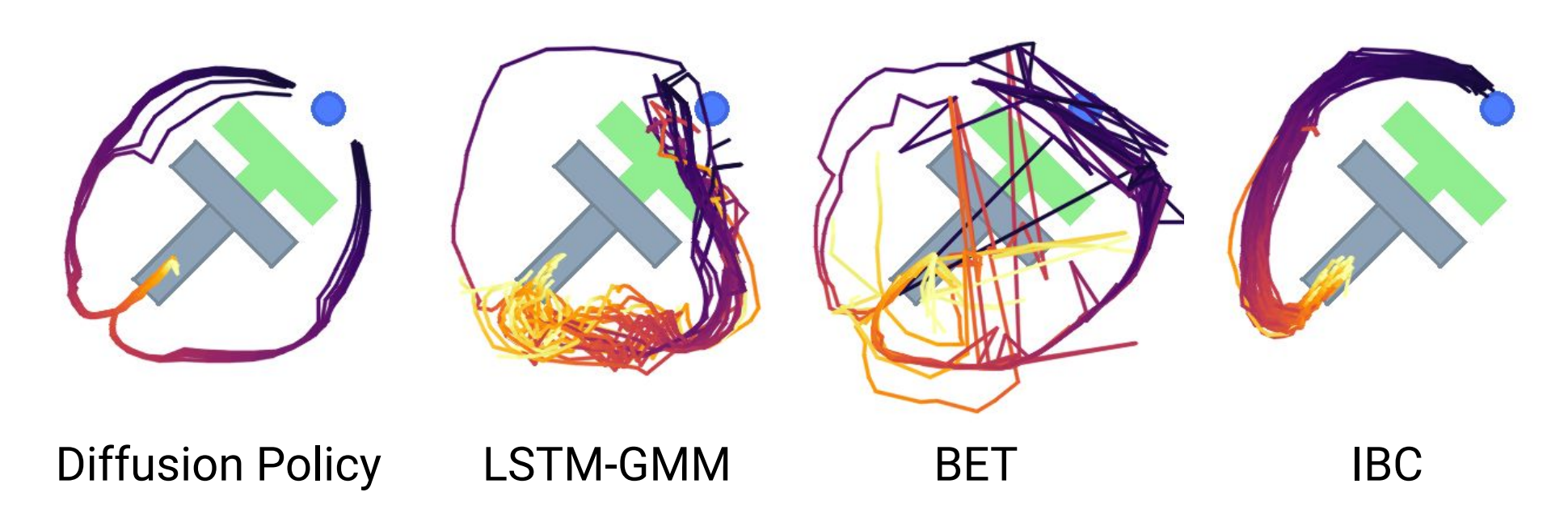
然而,如果序列中的每个动作被预测为独立的多模态分布(如在BC-RNN和BET中所做的那样)。连续动作可能会从不同模式中提取出来,并导致两个有效轨迹之间交替出现抖动动作
However, suppose each action in the sequence is predicted as independent multimodal distributions (as done in BCRNN and BET). In that case, consecutive actions could be drawn from different modes, resulting in jittery actions that alternate between the two valid trajectories. - 对于空闲动作的鲁棒性:当演示暂停并导致相同位置或接近零速度的连续动作序列时,则会发生空闲行为。这在远程操作等任务中很常见
然而,单步策略容易过度适应这种暂停行为。例如,在实际世界实验中使用BC-RNN和IBC时经常会卡住,未删除训练数据集中包含的空闲行为(BC-RNN andIBC often get stuck in real-world experiments when the idleactions are not explicitly removed from training)
// 待更
1.3.2 扩散模型在训练中的稳定
隐式策略使用基于能量的模型(EBM)表示动作分布(An implicit policy represents the action distribution using an Energy-Based Model (EBM)),如下公式6所示:

其中
是一个难以处理的归一化常数(相对于a)
为了训练用于隐式策略的EBM,使用了infonce风格的损失函数,它相当于公式6的负对数似然

在实践中,负采样的不准确性已知会导致EBMs的训练不稳定[11,48]
扩散策略和ddpm通过建模公式6中相同动作分布的得分函数[46],完全回避了
的估计问题:

因此,扩散策略的推理过程(公式4)和训练过程(公式5)都不涉及对
的评估,从而使扩散策略的训练更加稳定
第二部分 VINN
// 待更