环境介绍
- 用的mmyolo0.6.0导出的yolov7,如果导出模型有问题,参考我之前的文章。
- 我的数据集只有两个类别。
- 下图是我模型的输入输出。
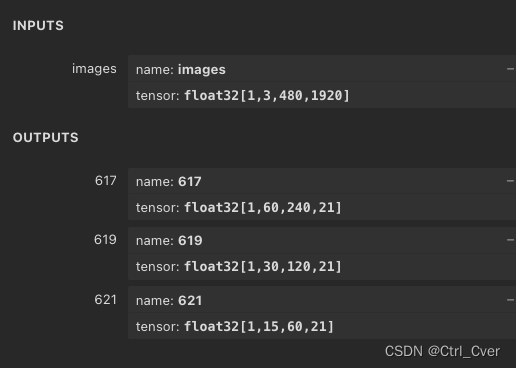
因为需要在边缘设备推理,所以先用python写一遍onnx的后处理。代码如下:
import onnxruntime
import numpy as np
import cv2
import os
import tqdm
from torchvision.ops import nms
import torch
onnx_model_path = 'work_dir/best_coco_bbox_mAP_epoch_190.onnx'
sess = onnxruntime.InferenceSession(onnx_model_path)
anchor8 = np.loadtxt('anchor_60_240.txt')
anchor16 = np.loadtxt('anchor_30_120.txt')
anchor32 = np.loadtxt('anchor_15_60.txt')
strides = [8,16,32]
coco_classes = [
'person', 'soccer'
]
def softmax(x):
exp_x = np.exp(x - np.max(x))
return exp_x / exp_x.sum(axis=0, keepdims=True)
def sigmoid(x):
return 1 / (1 + np.exp(-x))
def get_color(idx):
idx += 3
return (37 * idx % 255, 17 * idx % 255, 29 * idx % 255)
def onnx_infer(img_path):
img = cv2.imread(img_path)
img = cv2.resize(img,(1920,480))
timg = img[...,::-1]
input_data = np.transpose(timg,(2,0,1))[None,...]
input_data = np.array(input_data/255,dtype=np.float32)
output_data = sess.run(['617','619','621'], {'images': input_data})
ans_bboxs = []
ans_score = []
ans_cat = []
for output,anchor,stride in zip(output_data,(anchor8,anchor16,anchor32),strides):
_,h,w,c = output.shape
pred = output.reshape(-1,c)
for i in range(h*w):
pix = pred[i]
shifty = (i//w)*stride
shiftx = (i%w)*stride
cur_anchor = anchor+[shiftx,shifty,shiftx,shifty]
for k in range(3):
xc = (cur_anchor[k][0]+cur_anchor[k][2])/2
yc = (cur_anchor[k][1]+cur_anchor[k][3])/2
wc = cur_anchor[k][2] - cur_anchor[k][0]
hc = cur_anchor[k][3] - cur_anchor[k][1]
xc_pred = (sigmoid(pix[k*7+0]) - 0.5)*2*stride+xc
yc_pred = (sigmoid(pix[k*7+1]) - 0.5)*2*stride+yc
w_pred = (sigmoid(pix[k*7+2])*2)**2*wc
h_pred = (sigmoid(pix[k*7+3])*2)**2*hc
bbox_score = sigmoid(pix[k*7+4])
if pix[k*7+5] > pix[k*7+6]:
cat_score = pix[k*7+5]
cat_ = 0
else:
cat_score = pix[k*7+6]
cat_ = 1
conf = sigmoid(cat_score)*bbox_score
ltx = xc_pred - w_pred/2
lty = yc_pred - h_pred/2
rtx = xc_pred + w_pred/2
rty = yc_pred + h_pred/2
if bbox_score > 0.1:
ans_bboxs.append([ltx,lty,rtx,rty])
ans_score.append(conf)
ans_cat.append(cat_)
keep = nms(torch.Tensor(ans_bboxs), torch.Tensor(ans_score), iou_threshold=0.5)
ult_bbox = np.array(ans_bboxs)[keep]
ult_score = np.array(ans_score)[keep]
ult_cat = np.array(ans_cat)[keep]
for bbox,conf,cat in zip(ult_bbox,ult_score,ult_cat):
x0,y0,x1,y1 = bbox
cv2.rectangle(img,(int(x0),int(y0)),(int(x1),int(y1)),get_color(int(cat)),2,2)
label = f'{coco_classes[int(cat)]}: {conf:.2f}'
cv2.putText(img, label, (int(x0),int(y0) - 5), cv2.FONT_HERSHEY_SIMPLEX, 0.5, get_color(int(cat)), 2)
cv2.imwrite(os.path.join('onnx_infer',img_path.split('/')[-1]),img)
if __name__ == '__main__':
data_root = 'mydemo/'
imgs = os.listdir(data_root)
for img in tqdm.tqdm(imgs):
if img.endswith('jpg') or img.endswith('png'):
onnx_infer(os.path.join(data_root,img))
anchor是在下面这个文件保存的:
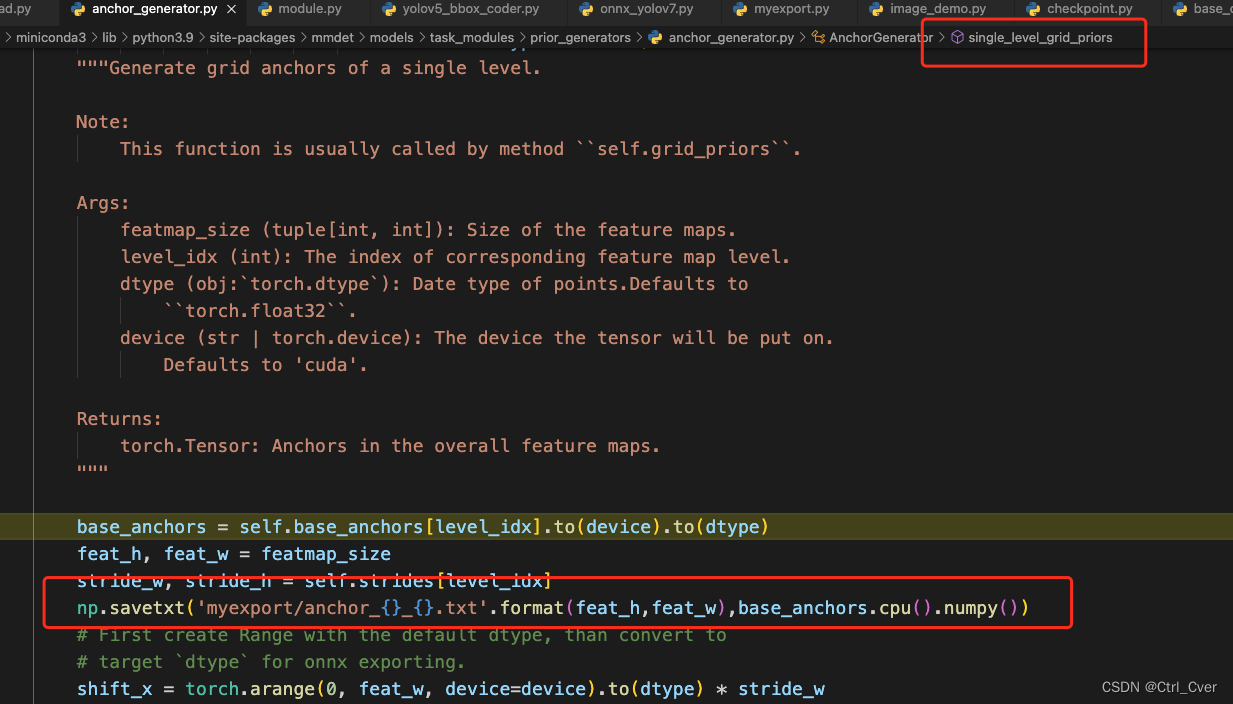